Four Essential
Steps for AI Project Success
Unlocking the True Potential of AI in Today’s Business Landscape
In today’s world, the presence of artificial intelligence (AI) is pervasive, with AI technology integrated into our vehicles, portable devices, and enterprise systems. According to IDC’s forecasts, organizations are projected to invest over $500 billion in AI hardware, software, and services in 2023, and this investment is growing at a robust rate of nearly 20% annually.1
AI technology is assuming an increasingly significant role in aiding business leaders in making critical decisions, offering the potential to unlock valuable insights that can differentiate businesses in product design, supply chain management, fraud detection, network security, and more.
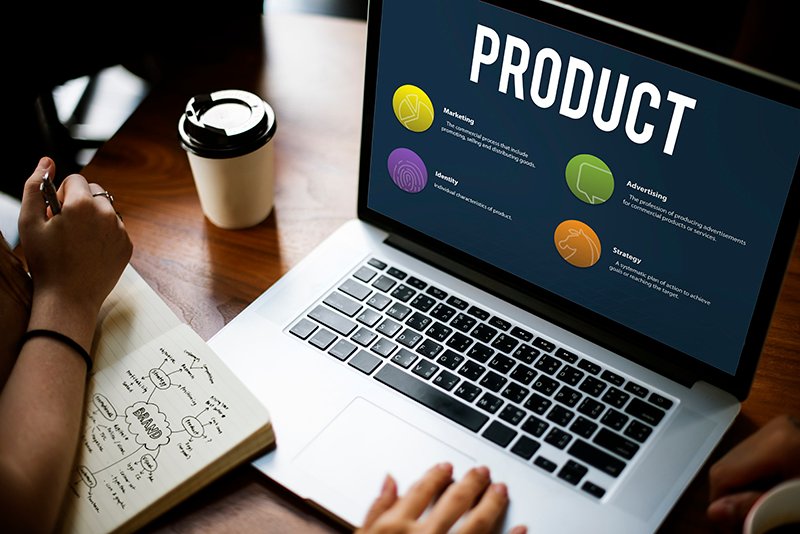
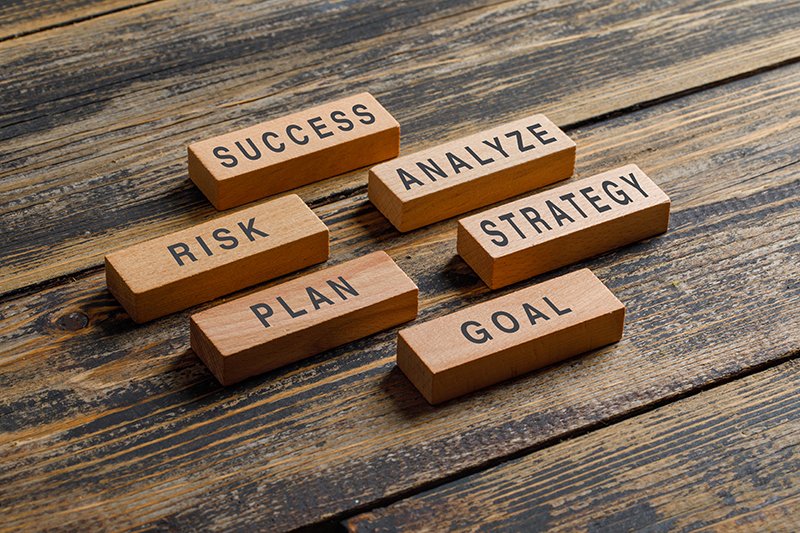
Despite the widespread adoption of AI, many enterprises are not realizing the full value of their AI investments, as up to 80% of AI models developed never see operational deployment.2 Various challenges contribute to this issue, as noted by Arti Garg, a distinguished technologist at Hewlett Packard Enterprise. One common challenge is the inaccuracy of AI models in making predictions for specific business decisions. Even when models yield valuable insights that align with organizational needs, constraints in resources and resistance to necessary organizational or technological changes can hinder deployment. Additionally, some AI models may not address problems critical enough to justify the deployment effort, often due to insufficient involvement of domain experts in the early stages of development.
However, Garg offers a set of steps to ensure the successful creation of AI solutions that unlock insights driving informed business decisions.
1. Ensure access to the required data
AI solutions offer the potential to extract valuable insights through data utilization. However, the initial step involves ensuring access to a sufficient quantity of the appropriate data, which must also be readily available, sanitized, and compliant with existing regulations. It is not uncommon for data scientists to construct models based on historical data, only to realize that it may not accurately represent the new data entering the system, as noted by Garg.
Moreover, this data may be compartmentalized, controlled by various segments within your organization. In cases where the data encompasses personally identifiable information (PII), financial documents, or health records, it may be constrained by privacy regulations or the data sovereignty rules set forth by the European Union. The data may also lack the timeliness required to generate insights within the necessary decision-making timeframe or could be cost-prohibitive to clean and incorporate.
Garg emphasizes the importance of determining the data realistically accessible within a production environment and aligning it with the timeline for generating business insights. Additionally, it may be necessary to incorporate the costs associated with data acquisition and ingestion into your budget and deployment schedule.
2. Engage in collaboration with essential stakeholders
In our society, we often glorify the solitary genius, toiling away in the lab and suddenly having a ‘eureka’ moment. However, the most effective AI solutions are the result of collaborative efforts. This is because multiple components need to harmonize, not just the model itself. Data scientists must collaborate with their IT and business counterparts to grasp existing business processes and tools, according to Garg.
“It’s relatively straightforward for an individual data scientist to create a prototype or proof of concept on their own,” she notes. “However, as you transition to full-scale production, numerous other stakeholders become involved, and predictions might lose their value if they’re not integrated into a broader decision-making framework. There may also be compliance requirements or internal best practices to consider. When embarking on an AI project, it’s imperative to identify the relevant stakeholders and involve them in the process.”
If the solution necessitates dismantling existing systems and replacing them, Garg advises that confidence in the AI solution’s ability to address problems better than previous methods is crucial.
3. Develop a model that effectively addresses genuine business challenges
“All too often, AI models generate insights that ultimately lack a significant impact on an organization’s success. Projects should demonstrate a measurable return on investment to progress,” remarks Garg. “Many AI projects may not provide sufficient value to your business. You must ask yourself: are you genuinely addressing the core problem that needs solving? Constructing a mathematical model for data interpretation is one thing, but transforming the decision-making process within a business is a completely different challenge.”
This means that, even when working with the same data set, your AI solution may be oriented toward diverse objectives. For instance, when developing an AI solution using customer service data, some organizations might prioritize reducing customer churn, while others aim to enhance customer service ratings or expand their reach to underserved demographics. In many cases, the underlying goal boils down to the metrics most closely associated with business value: cost and efficiency. For example, the implementation of AI in supply chain management has been demonstrated to reduce logistics costs by 15%, lower inventory levels by 35%, and improve service levels by 65%.3 AI-enabled document processing has enabled mortgage lenders to expedite loan closures by 12 days and slash the duration of their know-your-customer verification processes from two days to just five minutes.
4. How Iozera fills gaps in AI expertise
Even if you have a mature AI development team, you can use help working with IT to deploy their models to your broader organization. Iozera can provide tools and technologies that help you overcome some of your IT challenges, such as moving from a prototype built by a single data scientist to a more sophisticated production deployment that can be integrated with other, legacy capabilities, Garg says. At the same time, Iozera offers professional services that work with you at different stages of maturity to help you build your AI strategies and incorporate them into existing business processes. “Technology is usually the easy part,” Garg says. “Getting buy-in from the people involved in these processes can be a bigger and more difficult barrier to overcome.”
1 “IDC Forecasts Companies to Increase Spend on AI Solutions by 19.6% in 2022,” IDC, February 2022
2 “Models Are Rarely Deployed: An Industry-wide Failure in Machine Learning Leadership,” KDnuggets, January 2022
3 “Succeeding in the AI supply-chain revolution,” McKinsey, April 2021